Abstract
Vulnerability to climate change is a product of biophysical and social dynamics. Assessments of community or regional vulnerability, however, often focus on quantitative infrastructure and environmental assessments, or qualitative assessments of a community's social dynamics and livelihood activities. A dearth of integrated quantitative assessments is a major barrier for decision makers who require quantitative outputs and indicators, which can measure where vulnerability is most severe and can be linked to climate projections. Our framework and analysis helps address such gaps by identifying variables to build climate change vulnerability indices, which we pilot here focusing on Inuit communities in the Canadian Arctic. We start with a systematic literature review of community-based vulnerability studies and assess relationships among 58 social and biophysical variables. We then use multiplex network analysis to determine how social and environmental variables interact among and within the key component of vulnerability: exposure, sensitivity, and adaptive capacity. We identify several structurally important variables that interact within and across the three dimensions of vulnerability. This method is transferable as an integrative means of understanding not only the direct causes of vulnerability, but also relations that are less tangible. The approach of multiplex network analysis can be a building block to ongoing development of vulnerability indices within the human dimensions of climate change field.
Export citation and abstract BibTeX RIS

Original content from this work may be used under the terms of the Creative Commons Attribution 3.0 licence. Any further distribution of this work must maintain attribution to the author(s) and the title of the work, journal citation and DOI.
Introduction
Climate change is widely recognized as one of the major challenges of this century. Many regions and communities are already experiencing the effects of climate change with shifts in precipitation patterns, loss of coastline, and increases in frequency of severe weather events [1]. To help communities prepare for these changes, vulnerability research seeks to identify and characterize the various interacting climatic and non-climatic factors which create susceptibility to harm [2, 3]. Community vulnerability case studies have been widely used for adaptation and disaster risk reduction planning, climate change cost analysis, and delineation of relationships between social and physical environments, contributing to a growing knowledge of the multiscale dynamics of vulnerability to climate change [4–6].
Community-level studies have been a central feature of vulnerability research, and many focus on social variables. There is, however, strong demand for integrated quantitative regional vulnerability studies that consider both social and physical variables [7] and demand by government officials for indicators to do so as outlined by Canada's Expert Panel on Climate Change Adaptation and Resilience Results [8]. The potential to link downscaled climate model data with vulnerability assessments makes such assessments even more desirable [9–12]. With over 25 years of vulnerability case studies and literature summarizing commonalities and trends throughout the field, there are enough data to begin moving beyond community-level analysis in heavily studied regions, although few methods have been proposed for upscaling integrated vulnerability case studies. Addressing this key methodology gap, we propose the use of multiplex network analysis to draw together data from vulnerability case studies and identify which variables are most influential for developing a regional vulnerability index.
Vulnerability has been conceptualized in several different ways among different discipline and study perspectives [13–17]. Two distinct frameworks of vulnerability appear throughout the scholarship—risk hazard or outcome-based approaches, and pressure and release or contextual vulnerability [15, 18–21]. Although both approaches fundamentally denote the susceptibility of a population to harm, the associated research questions and perspectives differ [16, 18, 20, 22]. Outcome-based vulnerability assessments focus primarily on biophysical exposure and assess vulnerabilities directly related to these exposures, while contextual vulnerability begins with human systems and examines what makes populations vulnerable to external conditions in the context of multiple stresses [18, 23]. This framing of social vulnerability is also prevalent within the natural hazards scholarship by which disasters are seen as products of social structures, not solely biophysical magnitude [24]. This can be expressed as:

where E, S, and AC refer to exposure, sensitivity, and adaptive capacity of a community or region [25]. Exposure is defined as a change in frequency, magnitude, or duration of biophysical interactions with human systems or other biophysical variables [26–28]. Sensitivity is defined as conditions that alter the amount of impact an exposure may have on a biophysical or human system [26, 29, 30], and adaptive capacity reflects the ability to address, plan for, or adapt to climate-related risks and take advantage of new opportunities [26, 31]. These three components of vulnerability are not mutually exclusive and the relationships between variables can be scale dependent and may vary depending on the system being assessed [26]. We use contextual vulnerability in this study because of the emphasis placed on social systems as mechanisms for reduction (adaptation) or exacerbation of vulnerability and the ability to capture feedback loops and cross-sectoral interactions.
While study approaches for contextual vulnerability vary throughout the literature, most community-based case studies begin by examining the state of vulnerability (health burdens, food insecurity, unrest) and then dissect the social and physical pathways and influences. When focusing on specific livelihood segments and locations, the classification and system mapping is often straightforward. However, as we develop a model for operationalizing vulnerability assessments for regions and transitioning from qualitative to quantitative representations, selection of appropriate variables, identification of system characteristics, and accounting for scale dependent variance becomes increasingly complex. Here, we apply network analytics as a means of characterizing how different socio-economic, environmental, and climatic indicators interact to create vulnerability.
Informed by Ford and Smit [26], we contend that relationships of E, S, and AC can overlap and therefore are best represented by a multiplex network approach, where objects in the network can have multiple types of relationships. Development of multiplex tools is relatively new [32], and their application to human-environment research is even newer [33]. Therefore, this study not only advances vulnerability scholarship, but also network approaches to human-environment research. In this paper, we apply network analysis to assess vulnerability in the Canadian Arctic, a region undergoing some of the most rapid changes in climate globally [34], with a focus on Inuit communities (see map in SP-1, available online at stacks.iop.org/ERL/13/104019/mmedia).
Methods
Framework and research approach
Moving from qualitative community-based vulnerability studies to a regional system map of vulnerability (a model that can be eventually linked to social and environmental projections), requires an iterative and transferable approach. We begin by visualizing the vulnerability framework in a way that promotes quantitative operationalization of a systems analysis.
Informed by existing vulnerability frameworks, we first subset the system into exposure (E), sensitivity (S), and adaptive capacity (AC). Since a given variable can influence multiple components of vulnerability (i.e. socio-economic status may influence both adaptive capacity and sensitivity), we ensure that each vulnerability component includes all variables; each component can be represented as a two-dimensional matrix of system variables that together form a multi-layered system of interactions (figure 1).
Figure 1. Conceptualization of vulnerability as a multiplex network. On the left, two-dimensional matrices represent the coding process (positive, negative or unknown/variable; blue, yellow and white respectively). Variables are also coded for membership in four sub-networks from Canada's Marine Coasts Assessment (top of figure) so that the total network can be subdivided to focus on individual sectors/segments. Membership to sub-indices is not mutually exclusive. On the right, the matrices are translated into networks. The gray circles represent vulnerability variables (called nodes) and the presence of E, S, and AC relationships are illustrated by lines (called edges). Multiplex metrics are then calculated to understand the extent to which individual variables contribute to E, S, and AC for an integrated understanding of vulnerability.
Download figure:
Standard image High-resolution imageNext, we used a systematic literature review [35, 36] of climate change vulnerability case studies conducted in the Canadian Arctic to select index variables and understand their relationships. Figure 2 illustrates the steps used starting with the systematic literature review and ending with stakeholder engagement to validate and refine our results. Because the current research builds on more than 16 years of work by our research group with local Inuit communities and regional decision makers [5, 26, 37, 38], underpinned by principles of community-based participatory research [39, 40], we have the advantage of implicitly integrating substantial local insight into our project. We therefore, aim to re-engage stakeholders at the end, to validate and refine our results. Community work is a major investment for both researchers and community members and some Inuit communities have already voiced concerns about research fatigue [40]. To reduce fatigue, we propose coming to communities with a set of analyses and research findings in hand that can catalyze conversations, which has proven fruitful in other places [41]. When generalizing our approach to situations that lack such longstanding relationships, initial community collaboration is likely an important first step, as indicated in figure 2. The results of this paper focus on the literature review and multiplex network analysis of vulnerability (i.e. steps 1 through 5, figure 2). Further reflections about stakeholder engagement are provided in the discussion.
Figure 2. The figure illustrates the steps taken to implement the system's network approach for vulnerability assessment in the Canadian Arctic. Steps 1–5 are detailed throughout the article and step 6 is suggested as an exploratory and validation phase, which can be an iterative process or an alternative starting point.
Download figure:
Standard image High-resolution imageSystematic literature review
We began the review in Web of Knowledge using the following syntaxes during the 1970–2017 period: (a) 'climat* chang*' AND 'Inuit*' n = 240 records; (b) 'climat* chang*' AND 'vuln* Inuit*' n = 83 records; (c) 'climat* chang*' AND 'adapt* Inuit*' n = 87 records and finally (d) 'res*' AND 'Inuit*' n = 39 records. We also searched gray literature not computed by index tools, including institutional reports, consultant reports, book chapters and conference proceedings. These searches were conducted using repository searches (e.g. openDOAR), reference lists, and consultation with team members. For the gray literature, the following syntaxes were used: (a) 'Inuit climate change' n = 588 records; (b) 'Inuit vulnerability' n = 437; (c) 'Inuit adaptation' n = 274 and finally 'Inuit resilience' n = 10 records. See SP-1 for further details.
The 1758 articles, book, book chapters and conference proceedings were reviewed and organized using a peer review tool for scrutinizing called Rayyan Systemic Reviews Platform [42] (see systematic literature review SP-1). This online platform helps expedite the initial screening of abstracts and titles using a process of semi-automation while incorporating a high level of usability [42]. Using this online tool, our team checked articles and agreed if they should be included for a complete review. Articles and gray literature were excluded from the full review process if they did not relate to Inuit systems vulnerability or connect Inuit lifestyle to human physical or environmental shifts. Papers that did not provide solid evidence linking climate change, socio-economic, and environment factors were also excluded. The final review included 155 documents.
Reviewing these materials initially identified 137 vulnerability variables. Many variables were considered sub-sets of larger categories. We therefore merged variables so that the resulting dataset (n = 58, table 1) would contain variables of a similar specificity (see SP-1 for initial variables). For example, we considered walrus and ringed seal (animals important to subsistence food systems) to be part of variable called 'ice-flow animals'.
Table 1. Variables (n = 58) included in the Inuit vulnerability system grouped by major category. Acronyms are provided and used in figures 3 and 4.
Categories | Variables | Acronym | Categories | Variables | Acronym |
---|---|---|---|---|---|
Weather | Extreme weather event | XWtr | Biophysical environment (Ecosystems) | Permafrost | Prm |
Fog | Fog | Eustatic sea level rise | SLev | ||
Precipitation | Prcp | Native ice-flow animals | WlfI | ||
Temperature | Tmp | Native marine animals | WlFM | ||
Wind direction variability | WDir | Native plants | Plts | ||
Wind speed | WSpd | Native terrestrial animals | WlfT | ||
Hazards | Coastal erosion | Ersn | Sea ice | SIce | |
Floods | Fld | Health | Mental health | HthM | |
Slope failure | Slp | Physical health | HthP | ||
Storm surge | SSrg | Commercial transportation | Air transportation | ArTr | |
Infrastructure | Public buildings and roads | Publ | Overland transportation | LnTr | |
Airport infrastructure | Arp | Shipping | ShTr | ||
Energy availability | Eng | Public Services | Health care | HthC | |
Ports | Prt | Education quality and quantity | Edu | ||
Telecommunications | Telc | Emergency response | EmRs | ||
Waste management | Wst | Natural resource management | NRM | ||
Housing | Housing quality | HsQt | Risk management and future planning | RMP | |
Housing quantity | HsQl | Culture and Language | Arts and traditional equipment/clothing production | Trd | |
Economy | Cost of living | Cost | Environmental knowledge and skills | EvKS | |
Fishing (commercial) | FshC | Food sharing and social networks | FdShr | ||
Informal income | Inflc | Indigenous language strength | Lang | ||
Nat. resource extraction | NREx | Water Security | Water access | WtrAc | |
Relative poverty | Pov | Water quality | WtrQl | ||
Tourism | Trsm | Water quantity | WtrQt | ||
Wage income | WgIc | Personal Land Travel | Access to equipment | Eqp | |
Food Security | Country food quality | CnQt | Travel on the ice | TrvI | |
Country food quantity | CnQl | Travel on the land | TrvL | ||
Food access | FdAc | Travel on water | TrvW | ||
Store-bought food quality | StrQl | ||||
Store-bought food quantity | StrQt |
Among the 58 merged variables identified in the literature review, we coded the effects of theoretically changing the magnitude or quality of any given variable on other variables. Coding used a nominal and qualitative classification and was based on our detailed reading of the literature and subsequent understanding of biophysical and socio-political dynamics in the region. We coded effects as positive (an increase/decrease in 'A' has the same effect on 'B'), negative (an increase/decrease in 'A' has the opposite effect on 'B'), or variable (the relationships could be highly contextual, exhibit an inflection point, or demonstrate some other behavior). Positive, negative, and variable effects were considered for each vulnerability component (i.e. E, S, and AC) and each could have different interaction types (i.e. increasing 'A' could increase 'B' when considering sensitivity, but decrease 'B' when considering exposure). We only considered direct effects where, for example, changing 'A' directly effects 'B.' Situations where changing 'A' affects 'B' which then affects 'C' would be coded as two direct effects, 'A -> B' and 'B -> C', and not 'A -> C'. The network is directed and feedback between two variables 'A' and 'B' is permitted and coded as two relationships ('A -> B' and 'B' -> 'A'). Two researchers independently conducted all coding and then codes were checked for agreement; discrepancies were reviewed and discussed by the research team and recoded following a consensus decision.
Lastly, we categorized variables into four sub-networks as used in Canada's Marine Coasts Assessment [5] to conduct in depth analysis of specific dimensions of Inuit life. The four sub-networks are detailed in SP-3 and include the following sectors: (S1) infrastructure and transportation, (S2) business and economy, (S3) health and well-being, and (S4) culture-education and subsistence-harvesting.
Network analysis
We use network analytics to analyze variables that influence exposure, sensitivity, and adaptive capacity. A network approach is logical because it analyzes the relationships among variables and vulnerability is fundamentally a relational concept; vulnerability is produced by the interaction of various social and ecological factors [26]. A network consists of an assemblage of units, called nodes in network science, that are connected by edges. Nodes in our network represent the 58 variables identified in the literature review. Positive, negative, and variable effects were represented as edges, and each vulnerability component (E, S, and AC) constitutes a network layer, or one of multiple possible relationships in a multiplex network. We then analyzed a variable's role based on network structures. For example, a highly connected variable would be more central to understanding vulnerability than a variable with few connections. Such diagnostic approaches are commonly used for guiding resource management programs (e.g. as reviewed by [43]) as well as understanding policy interactions [44]. Diagnostic application to economic vulnerability also exist (e.g. vulnerably to peak oil [45]).
A network perspective often focuses on structural relationships, or the number and arrangement of nodes and edges [46]. For example, a single state agency that holds a governance network together, forming a network with a central hub and few connections among other nodes, will have significant influence over the network. If the agency is inefficient, all communications between parties will be slowed. If the agency dissolves, the network falls apart. The quality of actors (nodes) and relationships (edges) are also important in a network. When a central agency is trusted and all organizations agree on common objectives, a centralized network can be very efficient [47, 48]. A structural perspective does not ignore node and edge function and quality. It does however, recognize that real and potential function can be inferred by structural patterns that can then be further contextualized within specific cases as needed.
A fundamental structural property of any node in a network is connections to other nodes [46]. The number of connections is called a node's degree centrality (CD). The higher a node's degree centrality, the more important it is in the network from a structural perspective. In a multiplex case, however, two nodes may have the same total number of edges but play very different roles or function in the network. For example, consider a node 'A' with nine exposure edges and a node 'B' with nine edges spread evenly across the E, S, and AC categories. Despite having the same number of edges, the two nodes clearly play different roles in the network, the exact nature of which is contextual to the particular case. Node 'B' in the previous example is said to be more multiplex [49]. Analyzing the degree centrality and multiplex nature of vulnerability components helps untangle the complex web of interactions that create the coupled human-environment phenomena of vulnerability [16].
To understand how multiplex a node is, we calculate its participation coefficient (Pi) which is a measure of how evenly a node's edges are distributed among different categories. Following Battiston et al [49] who expanded the concept of single layer network participation [50] to multiplex networks, we consider Pi to be zero when a node has edges of only one kind (e.g. only exposure edges). Pi equals one when a node has an equal number of edges among all the categories in question (e.g. 3 E, 3 S, and 3 AC). Formally, Pi is defined as follows [49]:
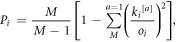
where M = the number of layers in the network (i.e. E, S, and AC), ki = a node's degree centrality for each of a layers, and oi = a node's total degree centrality across all network layers, also known as its total overlapping degree.
Following Battiston et al [49], we also consider Z-scores, which normalize the total overlapping degree to allow comparisons of networks of different sizes.

where 〈o〉 = the average overlapping degree of all nodes in the system and σo = the standard deviation. Unlike Pi, which is bound by 0 and 1, the Z-score is not bounded and its magnitude and range illustrate the variability of total degree overlap in the system.
Our vulnerability network is directed, meaning that edges did not have to be reciprocal; there could be an edge from i to j in layer a, but not from j to i. Degree centrality scores include edges to and from a node (i.e. total or freeman's degree centrality). When calculating centrality, Z-scores and participation we unweighted the data and only edge presence or absence was considered for these metrics. Our objective was to provide an initial coarse-grained diagnostic of vulnerability. We then analyzed the weighted data to understand system feedbacks using network diagrams and edge frequency counts. Further details and example code for calculating multiplex Pi, Z-scores, and total overlapping degree are provided in SP-2.
Results
Multiplex participation analysis
Nodes in the network vary greatly in terms of how multiplex they are (table 2, min and max Pi). No node, however, is perfectly multiplex or participating only within a single layer (0 < Pi < 1.00, table 1). The most multiplex variables in the whole vulnerability system (figure 3) includes travel on ice (Pi = 0.98), ports (Pi = 0.98), water quality Pi= 0.97), public buildings and roads (Pi = 0.96), shipping (Pi = 0.95) and travel on land (Pi = 0.95), implying that these are cross cutting variables that play an integral role in the whole vulnerability system.
Table 2. Descriptive statistics of multiplex scores accords all nodes (n = 58) in the entire network.
Participation coefficient (Pi) | Total overlapping degree (oi) | Normalized total overlapping degree (z) | |
---|---|---|---|
Min | 0.19 | 14.00 | −1.24 |
Max | 0.98 | 85.00 | 3.49 |
Mean | 0.77 | 32.62 | 0.00 |
Median | 0.75 | 30.50 | −0.14 |
Standard deviation | 0.13 | 14.90 | 0.99 |
Figure 3. Multiplex vulnerability network plot considering the whole vulnerability system. The y-axis Z represents the normalized total overlapping degree centrality. The x-axis Pi measures the distribution of edges among the different vulnerability dimensions E, S and AC. For clarity, abbreviated variables names are listed. Full names are available in the list in the right side of the figure.
Download figure:
Standard image High-resolution imageA different set of nodes has the highest total overlapping degrees: cost of living (oi = 85), poverty (oi = 77), wage income (oi = 71), natural resource management-planning (oi = 64) and extreme weather events (oi = 62). These variables concomitantly have medium-high participation scores (Pi) in the range of 0.64–0.78 indicating that they too are important cross cutting issues affecting Inuit vulnerability to climate change, though not the most multiplex. Within the network, variable-undetermined relationships are the most frequent relationships (n positive edges = 500, n negative edges = 460, n variable edges = 932). Inuit cost of living, for example has a total overlapping degree (oi) for variable-undetermined relationships of 76 and only nine positive and negative relationships combined. Cost of living is relatively multiplex (Pi = 0.75) and structurally important (oi = 85) for understanding Inuit vulnerability to climate change, but its specific interactions with other variables are uncertain illustrating a challenge when trying to parameterize a total vulnerability index. Any index of Inuit vulnerability should include a sensitivity analysis to a variable with multiplex scores such as those found in cost of living. The index might be calibrated under several scenarios or assumptions about a variable such as cost of living to present a range of results to aid decision makers.
The multiplex analysis condenses several dimensions of information into a single metric providing a powerful overview of the entire system. This analytical power, however, should not overshadow the merit of simultaneously learning through the lens of a more detailed analysis of each layer. For this reason, we further explore and dissect the whole vulnerability system into the three vulnerability layers of exposure, sensitivity and adaptive capacity (figure 4).
Figure 4. The network figure illustrates (a) exposure, (b) sensitivity and (c) adaptive capacity layers for the whole vulnerability system. Node size is proportional to the total number of network connections (Freeman's degree centrality, CD). Node colors represent the 13 category groups (see legend at bottom). Blue lines are positive relationships (an increase / decrease in 'a' causes the same change in 'b'). Red lines are negative relationships (an increase / decrease in 'a' causes the opposite change in 'b'). Black dashed lines are variable/undetermined relationships (outcome is contextual and/or a non-linear relationship). For clarity, only variable names discussed in the text are shown. See SP-3 for graphics with all variables labeled.
Download figure:
Standard image High-resolution imageInuit exposure, sensitivity and adaptive capacity to climate change
Inuit exposure to climate change
In the exposure layer, extreme weather has the largest degree centrality (CD = 27, figure 4, table 3) and would therefore affect many variables within the Inuit vulnerability system. Temperature is the second most central variable (CD = 24) and is expected to increase with climate change, leaving the region more prone to disasters such as floods (CD = 24) or storm surges (CD = 20) [51, 52]. Combining quantitative metrics with qualitative systems understanding can help parameterize a vulnerability model. For example, sea ice, another highly central variable (CD = 17), has many outgoing positive relationships (blue arrows, figure 4) to other variables that influence traditional food systems including food security, access, and animal resource abundance. Traditional activities have been shown to improve Inuit physical and mental health [53, 54] and therefore a variable like sea ice is not only structurally important in the network, but essential for Inuit well-being. Sea ice extent could serve as a key indicator variable within a vulnerability index.
Table 3. Names and Freeman's degree centrality (CD) scores of the top 10 rank ordered variables within each vulnerability layer (E, S, AC). The same nodes (n = 58) are present in each layer, but the number of relationship varies. In the table, management is abbreviated mgnt.
Rank order | Exposure | Sensitivity | Adaptive capacity |
---|---|---|---|
1 | Extreme weather events (CD = 27) | Relative poverty (CD = 48) | Wage income (CD = 49) |
2 | Temperature (CD = 24), | Cost of living (CD = 43) | Cost of living (CD = 42) |
Floods (CD = 24) | |||
3 | Storm surge (CD = 20) | Extreme weather events (CD = 35) | Risk mngt. and future planning (CD = 40) |
4 | Wind speed (CD = 19) | Natural resource mngt. (CD = 24) | Relative poverty (CD = 29) |
5 | Sea ice (CD = 17), | County food quantity (CD = 23) | Physical health (CD = 25) |
Coastal erosion (CD = 17) | |||
6 | Slope failure (CD = 16) | Precipitation (CD = 22), | Tourism (CD = 23), |
Wage income (CD = 22) | Mental health (CD = 23), | ||
Informal income (CD = 23) | |||
7 | Precipitation (CD = 13), | Temperature (CD = 21) | Food sharing and social networks (CD = 21) |
Eustatic sea level rise (CD = 13) | |||
8 | Permafrost (CD = 9), | Coastal erosion (CD = 20), | Arts and traditional equipment/clothing production (CD = 20), |
Travel on ice (CD = 9) | Fishing (commercial) (CD = 20) | Environmental knowledge and skills (CD = 20) | |
9 | Fog (CD = 8), | Floods (CD = 19), | Natural resource extraction (CD = 19) |
Overland transportation (CD = 8), | Country food quality (CD = 19) | ||
Public buildings and roads (CD = 8), | |||
Ports (CD = 8) | |||
10 | Travel on land (CD = 7) | Tourism (CD = 18), | Emergency response (CD = 17), |
Storm surge (CD = 18), | Indigenous language strength (CD = 17) | ||
Risk mngt. and future palming (CD = 18) |
Inuit sensitivity to climate change
Within the sensitivity layer, there are numerous variable-undetermined relationships, especially concerning the socioeconomic, cultural and well-being aspects of Inuit. It is logical that these variables have many variable-undetermined relationships; they are driven by people's actions, governance and the idiosyncrasies of specific regions and not necessarily reducible to simple direct relationships. Relative poverty has the highest degree centrality (CD = 48, figure 4, table 3). Other nodes with high degree centrality include cost of living (CD = 43), extreme weather events (CD = 35), and natural resource management (CD = 24). The large number of variable-undetermined edges indicate that it is not always possible to associate a given driver of change to perceived or documented consequences; there will be complex cumulative impacts of different drivers and trade-offs. This uncertainty highlights the need to calibrate vulnerability models at a local scale. Course grained assessments, as presented here, can help tease out assumptions and identify areas needing further research.
Inuit adaptive capacity to climate change
The adaptive capacity layer has several variable-undetermined and positive relationships. Wage income, with the highest degree centrality (CD = 49, figure 4, table 3), has high structural importance followed by cost of living (CD = 42), risk management and future planning (CD = 40) and natural resource management (CD = 34). Natural resource management and risk management, both of which are highly central, are affected by a wide range of local, regional, and national programs and capacities [55]. The vulnerability index will need to be calibrated for local variations in these important variables.
Discussion
Quantitative vulnerability indices are an important tool for adaptation planning [56]. Indicator variables should be easily applicable, measurable, accessible, transferable, and non-redundant [57]. While uncertainty in climate models and social and economic projections makes predicting future vulnerability challenging, vulnerability indices, nevertheless, provide a foundation for integrating future climatic and socio-economic drivers of vulnerability. Our systematic literature review and multiplex analysis advances vulnerability indicator creation by integrating social and biophysical variables derived from both community-based studies and large-scale regional reports. We also provide a methodology for identifying key indicator variables based on their centrality within a systems framework of vulnerability and ability to crosscut the three vulnerability components of exposure, sensitivity, and adaptive capacity.
In our analysis, cross cutting, multiplex variables, such as cost of living, poverty and wage income will play a vital role in future vulnerability index creation. Context, however, is important to consider when parameterizing. For example, tourism was highly central in S and AC dimensions. While potently a source of income (and possible adaptive capacity), studies have shown that tourism can be maladaptive when not properly integrated into local economies [58], so the effects of this variable must be evaluated carefully. Several nodes were less multiplex than expected, such as access to education, knowledge of traditional languages and health care. Low multiplex scores by no means challenge the established importance of these variables in the literature. Simply, these variables, which primary interact within a single vulnerability layer, may be more straightforward to parameterize in an index.
Our results provide important assessment of variables contributing to Inuit vulnerability. A few limitations and potential future expansions are worth noting however. First, several highly multiplex and central variables are categorically related such as poverty, costs of living, and wage income. These variables, however, cannot be treated as surrogates. Raising wages will have little effect on a person's life if the cost of living rises. Both variables must be considered, though perhaps could be calibrated into a living wage index. Our perspective is that the network analysis should always be paired with expert opinion when crafting vulnerability indices to account for such nuanced relationships and interdependences among nodes.
Second, our analysis uses several very powerful network metrics including multiplex participation, Z-scores, and degree centrality. We unweighted the network when calculating most metrics but included weights when considering edge counts and visual interpretation. Future expansions of our work might take advantage of other network metrics (see [59] for a review). For example, average node strength accounts for the value or strength of all edges and inverse participation ratio (not to be confused with multiplex participation, Pi, which we analyzed) evaluates how evenly weights are distributed among the edges [60]. These metrics could help highlight variables known to be important to Inuit well-being and resilience, such as education and traditional skills, that did not score high in our multiplex participation analysis.
Third, we do not take geography into account. Our review pulls together numerous case studies and regional reports to synthesize a set of vulnerability variables. It is possible that some variables are more pressing in specific regions of the Canadian Arctic due to local variability in resources, environment, and socio-political organization. Expansion of our work might attempt to integrate a geographic weighting or regional membership into the network analysis [43].
Finally, since our analysis started with the literature review, our results only reflect variables captured in the literature. Fortunately, there is a strong tradition of vulnerability and adaptation research in the Canadian Arctic, providing a robust dataset to pull on. While no minimum number of local case studies are needed for the network analysis, case study availability could be a barrier in other locations; but our approach can be applied to any population or setting provided there is data. Data availability and reliability are not unique concerns to the network approach. They are a challenge for any synthesis method. Network analysis is actually rather robust to small datasets. The multiplex analysis could be conducted with as few as three nodes, the bare minimum that constitutes a network. (Recall, nodes in our analysis are vulnerability variables, not the case studies from which variables are distilled.) Where quality case studies are lacking, other data sources such as media reports, or stakeholder interviews could also be used to generate nodes for the network analysis.
Even with a rich case study literature, stakeholder engagement, as outlined in figure 2, is a fundamental step to validate findings and elicit additional important variables. While we have chosen to engage stakeholders with a set of results in hand to catalyze discussions, reflecting extensive previous research and consultations conducted by the team in the region, other situations may warrant starting with stakeholders. The network analysis might also be treated as one of several methods, such as focus groups or expert opinions, to identify variables.
Stakeholder involvement should not be taken lightly or done superficially. Beyond essential issues related to community-based research, such as cultural understanding, trust, integrating disparate worldviews, and considering what is realistic, feasible, and desired involvement by communities [40, 61], there are inherent challenges of depth and breadth when engaging stakeholders for a locally informed and regionally focused project. Our literature review and network analysis aim to support indicator development for the entire Canadian Arctic, an area comprised of 53 communities spread out over a vast area >2 million km2 and four regional governments, each with rules and norms for conducting research (SP-1). Time, logistics, and finances may inhibit consulting every community. Researchers will have to work hard to achieve a legitimate engagement plan that yields the right level of stakeholder input as there are likely no panacea approaches.
Conclusion
Our multiplex network approach provides a flexible, quantitative framework to evaluate exposure, sensitivity, and adaptive capacity variables while recognizing that these dimensions of vulnerability are not mutually exclusive. Our results can help select indicators from available census and other government data to create vulnerability indices. For instance, accessing fine-resolution, up-to-date data for highly central and multiplex variables would be a top priority when compiling vulnerability indices. We can further calibrate indicator models based on their positive and negative relationships. Undetermined effects will have to be scrutinized depending on how they behave in context specific situations. Our approach, however, is not without limitations. We recommend using the network approach as one of several methods to identify indicator variables and that any index be developed with adequate and necessary involvement and feedback from local communities, decision makers, and subject experts.
Acknowledgments
Several anonymous reviewers provided important comments that improved this manuscript. This project was supported by funding from the Social Sciences and Humanities Research Council of Canada (SSHRC), Canadian Institute of Health Research (CIHR), Transport Canada's Northern Transportation Adaptation Initiative, and ArcticNet.